- How will competitors react if we do X?
- Will distributors increase or decrease support?
- What are commodity prices likely to do?
Decisions based on observable, validated data are usually the best ones. When you have the data, use it. If you’re lucky enough to have the right data in the right quantities for the question at hand, then let your analytical scientists drive and put your instincts in the passenger seat long enough to watch and learn.
But when you don’t have the data and you can’t buy it or develop a clear proxy for it from some other source, you still need to know how to make the decision. Sometimes, you might need to actually make the data. That probably sounds heretical to many of you who’ve invested a great deal of money and energy in beefing up your analytical capabilities. But where the analytics leave off and the questions linger, we succeed or fail by the quality of our guesses.The one approach for developing data proxies we've used with good success is response modeling, a tool that can help you make better guesses by talking to people with the right experience.
Response modeling in its simplest terms, requires assembling a group of people in your organization whom you believe have the experience to make sound educated guesses on specific issues you want to track. The process involves walking the group through a series of structured question-and-answer sessions — essentially completing a response card — in which you ask each of them very specific questions that zero in on one or more areas of uncertainty.
You might ask a group to predict where a certain product is going to be 12 months from now, then ask them to break that prediction down on a month-by-month basis. Then you ask a series of questions designed to uncover the drivers of the outcome and the relationships between the variables. For example:
- What would happen to sales if we doubled our advertising?
- What would happen if we cut it in half?
- What if we see one or two competitors flood our space with similar products?
- Based on that situation, what would we see if we doubled our advertising spend? Cut it in half?
Example: If every manager were asked about the likely effect on profits if advertising were increased by 25%, it would produce a spectrum of possible outcomes from “no effect” (or maybe even “modest decrease”) to “modest increase” to “significant increase.” Those outcomes could be plotted on a curve to show the range of expected outcomes.
Now if we asked for expectations for a 25% decrease, we could also plot those. And if we continued both up and down to 50%, 75%, and 100% increases, as well as 50%, 75%, and 100% decreases, we’d have a pretty clear set of predictions that we could statistically translate into a response model.
If we wanted to get more complex, we could ask the same group to predict the outcome of simultaneously changing advertising spend and changing direct mail. Human beings with experience in the business will use their knowledge and intuition to develop individual best-guess outcomes. The matrix might look like this:
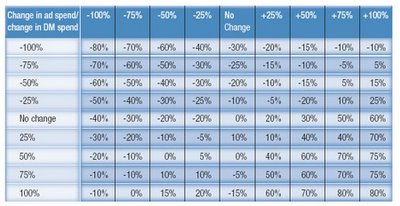
The model you create represents the collective tribal wisdom on a particular issue that might otherwise be tough to turn into a metric because you don’t have the data. Response models are really nothing more than a highly structured way of helping a management team direct its experience into an aggregated best guess. This may seem unpredictable, but in reality it helps identify the subtle relationships between actions and outcomes while removing some of the risk of any single individual being wildly wrong.
Every manager can form an opinion on the likely result of a certain action or inaction solely on the basis of their experience. The cumulative experience base within a company is often the most powerful untapped data source. Harnessing those individual perspectives into a collective view often provides tremendous insight helpful in making hard decisions. Of course, this approach is vulnerable to bad guessing by the entire group (which would be the Achilles heel of the company anyway), or even to sabotage by those who have an axe to grind against a certain form of spending. But if your group is diverse enough, it’s not hard to minimize these risks and improve the quality of the outcome.
No comments:
Post a Comment